Prof. Talia Gillis (Columbia) on the Fairness of Machine-Assisted Human Decisions
Talking law and economics at ETH Zurich - A podcast by ETH Center for Law & Economics
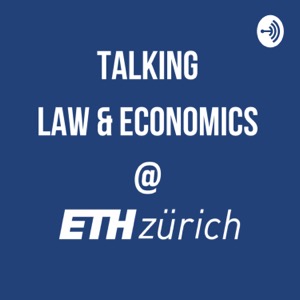
Categories:
When machine-learning algorithms are deployed in high-stakes decisions, we want to ensure that it leads to fair and equitable outcomes. However, many machine predictions are deployed to assist in decisions where a human decision-maker retains the ultimate decision authority. In this episode of the CLE's podcast series, Prof. Talia Gillis (Columbia) and Prof. Alexander Stremitzer (ETH Zurich) discuss Gillis' study "On the Fairness of Machine-Assisted Human Decisions" - joint with Bryce McLaughlin (Stanford) and Jann Spiess (Stanford) - on how properties of machine predictions affect the resulting human decisions. In their study, Gillis, McLaughlin and Spiess show in a formal model that the inclusion of a biased human decision-maker can revert common relationships between the structure of the algorithm and the qualities of resulting decisions. Specifically, they document that excluding information about protected groups from the prediction may fail to reduce disparities. Their results demonstrate more broadly that any study of critical properties of complex decision systems, such as the fairness of machine-assisted human decisions, should go beyond focusing on the underlying algorithmic predictions in isolation. Paper References: Talia Gillis - Columbia University Bryce McLaughlin - Stanford University Jann Spiess - Stanford University On the Fairness of Machine-Assisted Human Decisions https://arxiv.org/abs/2110.15310 Audio Credits for Trailer: AllttA by AllttA https://youtu.be/ZawLOcbQZ2w