Wikimedia MLOps // Chris Albon // Coffee Sessions #68
MLOps.community - A podcast by Demetrios
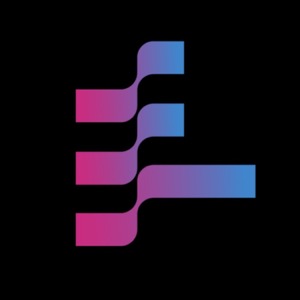
Categories:
MLOps Coffee Sessions #68 with Chris Albon, Wikimedia MLOps co-hosted by Neal Lathia. // Abstract // Bio Chris spent over a decade applying statistical learning, artificial intelligence, and software engineering to political, social, and humanitarian efforts. He is the Director of Machine Learning at the Wikimedia Foundation. Previously, Chris was the Director of Data Science at Devoted Health, Director of Data Science at the Kenyan startup BRCK, cofounded the AI startup Yonder, created the data science podcast Partially Derivative, was the Director of Data Science at the humanitarian non-profit Ushahidi, and was the director of the low-resource technology governance project at FrontlineSMS. Chris also wrote Machine Learning For Python Cookbook (O’Reilly 2018) and created Machine Learning Flashcards. Chris earned a Ph.D. in Political Science from the University of California, Davis researching the quantitative impact of civil wars on health care systems. He earned a B.A. from the University of Miami, where he triple majored in political science, international studies, and religious studies. // Relevant Links --------------- ✌️Connect With Us ✌️ ------------- Join our slack community: https://go.mlops.community/slack Follow us on Twitter: @mlopscommunity Sign up for the next meetup: https://go.mlops.community/register Catch all episodes, Feature Store, Machine Learning Monitoring and Blogs: https://mlops.community/ Connect with Demetrios on LinkedIn: https://www.linkedin.com/in/dpbrinkm/ Connect with Neal on LinkedIn: https://www.linkedin.com/in/nlathia/ Connect with Chris on LinkedIn: https://www.linkedin.com/in/chrisralbon/ Timestamps: [00:00] Introduction to Chris Albon [00:28] Do you sleep? :-) [02:43] ML at Wikimedia [09:27] Wikimedia workflow [15:00] Creating a repeatable process [19:11] Wikimedia element team size [20:47] Wikimedia workflow and hardware [23:56] Evaluating open source [29:20] Lacking in ML source tooling [33:11] Wikimedia's separate data platform [38:14] Abstractions [41:50] Experimentation aspect of getting models into production [44:05] Stack of Abstraction in ML [47:16] Chris' proudest model [49:10] How Wikimedia work with communities [55:24] Large language models [1:02:16] Beautiful vision [1:03:23] Wrap up