When Machine Learning meets privacy - Episode 6
MLOps.community - A podcast by Demetrios
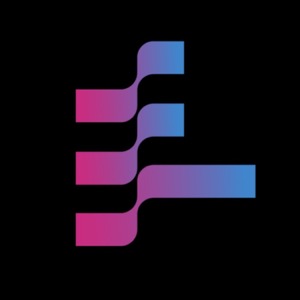
Categories:
**Privacy-preserving ML with Differential Privacy** Differential privacy is without a question one of the most innovative concepts that came around in the last decades, with a variety of different applications even when it comes to Machine Learning. Many are organizations already leveraging this technology to access and make sense of their most sensitive data, but what is it? How does it work? And how can we leverage it the most? To explain this and provide us a brief intro on Differential Privacy, I've invited Christos Dimitrakakis. Professor at University, counts already with multiple publications (more than 1000!!!) in the areas of Machine Learning, Reinforcement Learning, and Privacy. Useful links: Christos Dimitrakakis list of publications Differential privacy for Bayesian inference through posterior sampling Authors: Christos Dimitrakakis, Blaine Nelson, Zuhe Zhang, Aikaterini Mitrokotsa, Benjamin IP Rubinstein Differential privacy use cases Open-source differential privacy projects Open-source project for Differential Privacy in SQL databases