Tour of Upcoming Features on the Hugging Face Model Hub // Julien Chaumond // MLOps Coffee Sessions #48
MLOps.community - A podcast by Demetrios Brinkmann
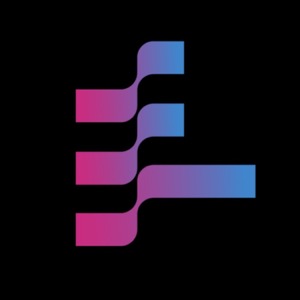
Categories:
Coffee Sessions #48 with Julien Chaumond, Tour of Upcoming Features on the Hugging Face Model Hub. //Abstract Julien Chaumond’s Tour of Upcoming Features on the Hugging Face Model Hub. Our MLOps community guest in this episode is Julien Chaumond the CTO of Hugging Face - every data scientist’s favorite NLP Swiss army knife. Julien, David, and Demetrios spoke about many topics including: Infra for hosting models/model hubs Inference widgets for companies with CPUs & GPUs (for companies) Auto NLP which trains models “Infrastructure as a service” // Bio Julien Chaumond is Chief Technical Officer at Hugging Face, a Brooklyn and Paris-based startup working on Machine learning and Natural Language Processing, and is passionate about democratizing state-of-the-art AI/ML for everyone. --------------- ✌️Connect With Us ✌️ ------------- Join our slack community: https://go.mlops.community/slack Follow us on Twitter: @mlopscommunity Sign up for the next meetup: https://go.mlops.community/register Connect with Demetrios on LinkedIn: https://www.linkedin.com/in/dpbrinkm/ Connect with David on LinkedIn: https://www.linkedin.com/in/aponteanalytics/ Connect with Julien on LinkedIn: https://www.linkedin.com/in/julienchaumond/ Timestamps: [00:00] Introduction to Julien Chaumond [01:57] Julien's background in tech [04:35] "I have this vision of building a community where the greatest people in AI can come together and basically invent the future of Machine Learning together." [04:55] What is Hugging Face? [06:17] "We have the goal of bridging the gap between research and production on actual production use cases." [06:45] Start of open-source in Hugging Face [07:50] Chatbox experiment (reference resolution system) - linking pronouns to the subjects of sentences [10:20] From a project to a company [11:46] "The goal was to explore in the beginning." [11:57] Importance of platform [14:25] "Transfer learning is an efficient way of Machine Learning. Providing your platform around change that people want to start from pre-trained model and fine-tune them into the specific use case is something that can be big so we built some stuff to help people do that." [15:35] Narrowing down the scope of service to provide [16:27] "We have some vision of what we want to build but a lot of it is the small incremental improvements that we bring to the platform. I think it's the natural way of building stuff nowadays because Machine Learning is moving so fast." [20:00] Model Hubs [22:37] "We're guaranteeing that we don't build anything that introduces any lagging to Hugging Face because we're using Github. You'll have that peace of mind." [26:31] Storing model artifacts [27:00] AWS - cache - stored to an edge location all around the globe [28:39] Inference widgets powering [27:17] "For each model on the model hub we try to ensure that we have the metadata about the model to be able to actually run it." [32:11] Deploying infra function [32:38] "Depending on the model and library, we optimize the custom containers to make sure that they run as fast as possible on the target hardware that we have." [34:59] "Machine Learning is still pretty much hardware dependent." [36:11] Hardware usage [39:04] "CPU is super cheap. If you are able to run Berks served with a 1-millisecond on CPU because you have powerful optimizations, you don't really need GPUs anymore. It's cost-efficient and energy-efficient." [40:30] Challenges of Hugging Face and what you learned [41:10] "It may sound like a super cliche but the team that you assembled is everything." [43:22] War stories in Hugging Face [44:12] "Our goal is more forward-looking to be helpful as much as we can to the community." [48:25] Hugging Face accessibility