"Real-Time" ML: Features and Inference // Sasha Ovsankin and Rupesh Gupta // MLOps Podcast #135
MLOps.community - A podcast by Demetrios Brinkmann
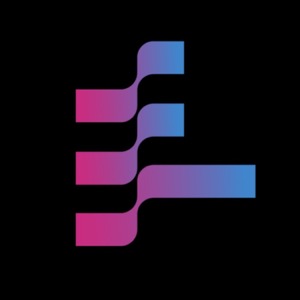
Categories:
MLOps Coffee Sessions #135 with Sasha Ovsankin and Rupesh Gupta, Real-time Machine Learning: Features and Inference co-hosted by Skylar Payne. // Abstract Moving from batch/offline Machine Learning to more interactive "near" real-time requires knowledge, team, planning, and effort. We discuss what it means to do real-time inference and near-real-time features when to do this move, what tools to use, and what steps to take. // Bio Sasha Ovsankin Sasha is currently a Tech Lead of Machine Learning Model Serving infrastructure at LinkedIn, worked also on Feathr Feature Store, Real-Time Feature pipelines, designed metric platforms at LinkedIn and Uber, and was co-founder in two startups. Sasha is passionate about AI, Software Craftsmanship, improvisational music, and many more things. Rupesh Gupta Rupesh is a Sr. Staff Engineer in the AI team at LinkedIn. He has 10 years of experience in search and recommender systems. // MLOps Jobs board https://mlops.pallet.xyz/jobs // MLOps Swag/Merch https://mlops-community.myshopify.com/ // Related Links --------------- ✌️Connect With Us ✌️ ------------- Join our slack community: https://go.mlops.community/slack Follow us on Twitter: @mlopscommunity Sign up for the next meetup: https://go.mlops.community/register Catch all episodes, blogs, newsletters, and more: https://mlops.community/ Connect with Demetrios on LinkedIn: https://www.linkedin.com/in/dpbrinkm/ Connect with Skylar on LinkedIn: https://www.linkedin.com/in/skylar-payne-766a1988/ Connect with Sasha on LinkedIn: https://www.linkedin.com/in/sashao/ Connect with Rupesh on LinkedIn: https://www.linkedin.com/in/guptarupesh Timestamps: [00:00] Sasha's and Rupesh's preferred coffee [01:30] Takeaways [07:23] Changes in LinkedIn [09:21] "Real-time" Machine Learning in LibnkedIn [13:08] Value of Feedback [14:24] Technical details behind getting the most recent information integrated into the models [16:53] Embedding Vector Search action occurrence [18:33] Meaning of "Real-time" Features and Inference [20:23] Are "Real-time" Features always worth that effort and always helpful? [23:22] Importance of model application [25:26] Challenges in "Real-time" Features [30:40] System design review on Pinterest [36:13] Successes of real-time features [38:31] Learnings to share [45:52] Branching for Machine Learning [48:44] Not so talked about discussion of "Real-time" [51:09] Wrap up