Practitioners Guide to MLOps // Donna Schut and Christos Aniftos // Coffee Sessions #82
MLOps.community - A podcast by Demetrios Brinkmann
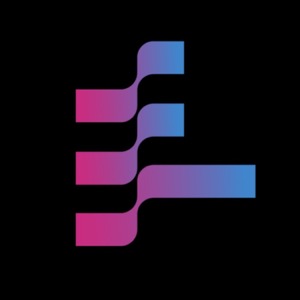
Categories:
MLOps Coffee Sessions #82 with Donna Schut and Christos Aniftos, Practitioners Guide to MLOps. // Abstract The "Practitioners Guide to MLOps" introduced excellent frameworks for how to think about the field. Can we talk about how you've seen the advice in that guide applied to real-world systems? Is there additional advice you'd add to that paper based on what you've seen since its publication and with new tools being introduced? Your article about selecting the right capabilities has a lot of great advice. It would be fun to walk through a hypothetical company case and talk about how to apply that advice in a real-world setting. GCP has had a lot of new offerings lately, including Vertex AI. It would be great to talk through what's new and what's coming down the line. Our audience always loves hearing how tool providers like GCP think about the problems customers face and how tools are correspondingly developed. // Bio Donna Schut Donna is a Solutions Manager at Google Cloud, responsible for designing, building, and bringing to market smart analytics and AI solutions globally. She is passionate about pushing the boundaries of our thinking with new technologies and creating solutions that have a positive impact. Previously, she was a Technical Account Manager, overseeing the delivery of large-scale ML projects, and part of the AI Practice, developing tools, processes, and solutions for successful ML adoption. She managed and co-authored Google Cloud’s AI Adoption Framework and Practitioners' Guide to MLOps. Christos Aniftos Christos is a machine learning engineer with a focus on the end-to-end ML ecosystem. On a typical day, Christos helps Google customers productionize their ML workloads using Google Cloud products and services with special attention on scalable and maintainable ML environments. Christos made his ML debut in 2010 while working at DigitalMR, where he led a team of data scientists and developers to build a social media monitoring & analytics tool for the Market Research sector. // Related links: Select the Right MLOps Capabilities for Your ML Usecase https://cloud.google.com/blog/products/ai-machine-learning/select-the-right-mlops-capabilities-for-your-ml-use-case Practitioner's Guide to MLOps white paper https://services.google.com/fh/files/misc/practitioners_guide_to_mlops_whitepaper.pdf --------------- ✌️Connect With Us ✌️ ------------- Join our slack community: https://go.mlops.community/slack Follow us on Twitter: @mlopscommunity Sign up for the next meetup: https://go.mlops.community/register Catch all episodes, blogs, newsletter and more: https://mlops.community/ Connect with Demetrios on LinkedIn: https://www.linkedin.com/in/dpbrinkm/ Connect with Vishnu on LinkedIn: https://www.linkedin.com/in/vrachakonda/ Connect with Donna on LinkedIn: https://www.linkedin.com/in/donna-schut/ Connect with Christos on LinkedIn: https://www.linkedin.com/in/aniftos/ Timestamps: [00:00] Introduction to Donna Schut and Christos Aniftos [05:52] Inspiration of Practitioner's Guide to MLOps paper [06:57] Model for working with customers [08:14] Where are we at MLOps? [10:20] Process of working with customers [11:30] Overview of processes and capabilities outlined in Practitioner's Guide to MLOps paper [16:16] Continuous Training maturity levels [22:37] Context about the discovery process [25:21] Disciplines and security mix tend to see [26:12] Is there a level up in maturity? [29:50] Success or failures that stand out [38:00] War stories [43:16] Internal study of qualities of the best ML engineers