MLOps Meetup #30 // Path to Production and Monetizing Machine Learning // Vin Vashishta - Data Scientist | Strategist | Speaker & Author
MLOps.community - A podcast by Demetrios
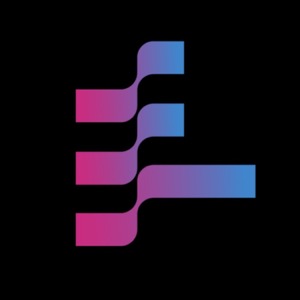
Categories:
The concept of machine learning products is a new one for the business world. There is a lack of clarity around key elements: Product Roadmaps and Planning, the Machine Learning Lifecycle, Project and Product Management, Release Management, and Maintenance. In this talk, we covered a framework specific to Machine Learning products. We discussed the improvements businesses can expect to see from a repeatable process. We also covered the concept of monetization and integrating machine learning into the business model. Vin is an applied data scientist and teaches companies to monetize machine learning. He is currently working on a ML based decision support product as well as my strategy consulting practice. Join our slack community: https://join.slack.com/t/mlops-community/shared_invite/zt-391hcpnl-aSwNf_X5RyYSh40MiRe9Lw Follow us on Twitter: @mlopscommunity Sign up for the next meetup: https://go.mlops.community/register Connect with Demetrios on LinkedIn: https://www.linkedin.com/in/dpbrinkm/ Connect with Vin on LinkedIn: https://www.linkedin.com/in/vineetvashishta/ Timestamps: [00:00] Intro to Vin Vashishta [01:33] Vin's background [05:04] Key problems when monetizing Machine Learning [07:00] How can we fix the key problems in monetizing Machine Learning [13:24] How can we go about creating that repeatable process? [16:17] There are all these data scientists aren't going to school and getting all these diplomas for data wrangling. Right? [17:12] How can you successfully envision that road mapping from the beginning of the process? [24:19] How can a Data Scientist be more proactive instead of just getting paid? [28:53] Have you figured out how to quickly estimate an order of magnitude when ROI questions arise? [31:48] Have you seen a company that has machine learning as its core product or have you seen some companies crash and burn? [34:39] How do you see the tooling ecosystem right now? And how do you see it in a few years? [38:24] And so how do you balance that when a lot of these tools have a lot of like, bleed and overlap? And so what does that look like? [42:40] Have you stumbled across organizations wanting to adopt AI without having the foundations such as data? [45:28] How can we convince human curators to do machine learning? [47:23] What are the three biggest challenges you've faced when monetizing the value of ML products. How did you overcome them? [50:25] How do you deal with people measuring costs and values?