ML Stepping Stones: Challenges & Opportunities for Companies // John Crousse // Coffee Sessions #67
MLOps.community - A podcast by Demetrios
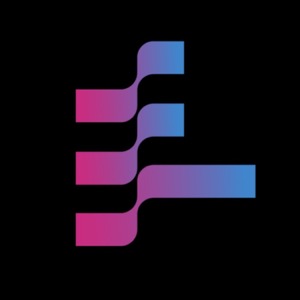
Categories:
MLOps Coffee Sessions #67 with John Crousse, ML Stepping Stones: Challenges & Opportunities for Companies co-hosted by Adam Sroka. // Abstract In this coffee session, John shares his observations after working with multiple companies which were in the process of scaling up their ML capabilities. John's observations are mostly around changes in practices, successes, failures, and bottlenecks identified when building ML products and teams from scratch. John shares a few thoughts on building long-term products vs short-term projects, on the important non-ML components, and the most common missing pieces he sees in today's ecosystem. John also elaborates on how those challenges and solutions can differ for different company sizes. // Bio John always liked CS/ML/AI but wasn't such a hot topic back then. He found opportunities to work on models in the Financial industry as a consultant from 2007 to 2017 then he went freelance to move outside of the financial industry, and focus on AI/ML. John likes to do things efficiently, and MLOps is the bottleneck, so he ended up spending more time on MLOPs than models lately. John finished his CS degree in 2007. // Relevant Links --------------- ✌️Connect With Us ✌️ ------------- Join our slack community: https://go.mlops.community/slack Follow us on Twitter: @mlopscommunity Sign up for the next meetup: https://go.mlops.community/register Catch all episodes, Feature Store, Machine Learning Monitoring and Blogs: https://mlops.community/ Connect with Demetrios on LinkedIn: https://www.linkedin.com/in/dpbrinkm/ Connect with Adam on LinkedIn: https://www.linkedin.com/in/aesroka Connect with John on LinkedIn: https://www.linkedin.com/in/john-crousse-31219b9 Timestamps: [00:00] Introduction to John Crousse [01:11] Main trends in Machine Learning [03:07] Symptoms of Machine Learning product [05:05] Proper product with limited resources [08:52] Going into production mindsets [11:22] Bottlenecks and challenges [14:55] Business case for Machine Learning or MLOps in small organizations [17:04] Gathering feedbacks best suited to product owners [19:14] More substantial role [20:11] Data factory [24:03] Delivery patterns or tech stacks [26:06] Bottleneck metrics [27:28] Concept of evaluation store [32:18] The biggest gap to bridge [34:42] Hindrance to people's development [35:23] "The last mile of the machine learning projects" [36:40] MLOps assessment survey [40:10] Who owns the product and path to recommend [41:34] Datamesh community [44:41] Tips on balancing between pure autonomy [45:58] Wrap up