How Explainable AI is Critical to Building Responsible AI // Krishna Gade MLOps // Meetup #53
MLOps.community - A podcast by Demetrios
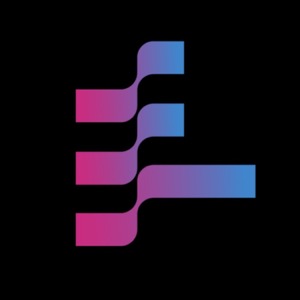
Categories:
MLOps community meetup #53! Last Wednesday we talked to Krishna Gade, CEO & Co-Founder, Fiddler AI. // Abstract: Training and deploying ML models have become relatively fast and cheap, but with the rise of ML use cases, more companies and practitioners face the challenge of building “Responsible AI.” One of the barriers they encounter is increasing transparency across the entire AI lifecycle to not only better understand predictions, but also to find problem drivers. In this session with Krishna Gade, we will discuss how to build AI responsibly, share examples from real-world scenarios and AI leaders across industries, and show how Explainable AI is becoming critical to building Responsible AI. // Bio: Krishna is the co-founder and CEO of Fiddler, an Explainable AI Monitoring company that helps address problems regarding bias, fairness and transparency in AI. Prior to founding Fiddler, Gade led the team that built Facebook’s explainability feature ‘Why am I seeing this?’. He’s an entrepreneur with a technical background with experience creating scalable platforms and expertise in converting data into intelligence. Having held senior engineering leadership roles at Facebook, Pinterest, Twitter, and Microsoft, he’s seen the effects that bias has on AI and machine learning decision-making processes, and with Fiddler, his goal is to enable enterprises across the globe solve this problem. ----------- Connect With Us ✌️------------- Join our Slack community: https://go.mlops.community/slack Follow us on Twitter: @mlopscommunity Sign up for the next meetup: https://go.mlops.community/register Connect with Demetrios on LinkedIn: https://www.linkedin.com/in/dpbrinkm/ Connect with Krishna on LinkedIn: https://www.linkedin.com/in/krishnagade/ Timestamps: [00:00] Thank you Fiddler AI! [01:04] Introduction to Krishna Gade [03:19] Krisha's Background [08:33] Everything was fine when you were doing it behind the scenes. But then when you put it out into the wild, we just lost our "baby." It's no longer under our control. [08:53] "You want to have the assurance of how the system works. Even if it's working fine or if it's not working fine." [09:37] What else is Explainability? Can you break that down for us? [13:58] "Explainability becomes the cornerstone technology to have in place for you to build Responsible AI in production." [14:48] For those used cases that aren't as high stakes, do you feel it's important? Is it up the foodchain? [18:47] Can we dig into that used case real fast? [22:01] If it is a human doing it, there's a lot more room for error? Bias or theories can be introduced and then they don't have a basis in reality? [23:51] Do you need these subject matter experts or someone who is very advanced to be able to set up what the Explainability tool should be looking for at first is it that plug and play and it will know it latches on to the model? [29:36] Does Explainable AI also entail Explainable Data. I see the point where Explainability can help with getting the insights about data after the model has been trained but should it be handled perhaps more proactively where you unbias the data before training the model on it? [32:16] As a data scientist, there are situations when the prediction output is expected to support a business decision taken by senior executives. In that case, when the Explainable model gives out a prediction that doesn't align with the stakeholder's expectations, how should one navigate through this tricky situation? [43:49] How are denen gram clustering for data explainability?