Robustness and generalization performance of Deep Learning Models on Cyber-Physical Systems
Industrial AI Podcast - A podcast by Robert Weber / Peter Seeberg - Thursdays
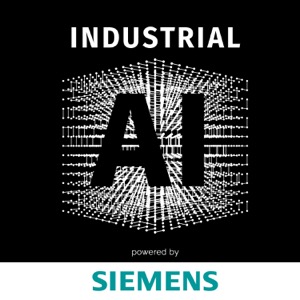
Categories:
Deep learning (DL) models have seen increased attention for time series forecasting, yet the application on cyber-physical systems (CPS) is hindered by the lacking robustness of these methods. Thus, this study evaluates the robustness and generalization performance of DL architectures on multivariate time series data from CPS. Peter Seeberg talked to the authors. Thanks for listening. We welcome suggestions for topics, criticism and a few stars on Apple, Spotify and Co. We thank our partner [Siemens ](https://new.siemens.com/global/en/products/automation/topic-areas/artificial-intelligence-in-industry.html) Olivers Paper: https://arxiv.org/abs/2306.07737 Thanks to Women in AI and Robotics ([more](https://www.womeninairobotics.de/)) PLEASE fill out the survey from Gabriel Krummenacher and the ETH Zurich [https://www.zuehlke.com/en/machine-learning](https://www.zuehlke.com/en/machine-learning) We thank our team: Barbara, Anne and Simon!