114 - Designing Anti-Biasing and Explainability Tools for Data Scientists Creating ML Models with Josh Noble
Experiencing Data w/ Brian T. O’Neill (UX for AI Data Products, SAAS Analytics, Data Product Management) - A podcast by Brian T. O’Neill from Designing for Analytics - Tuesdays
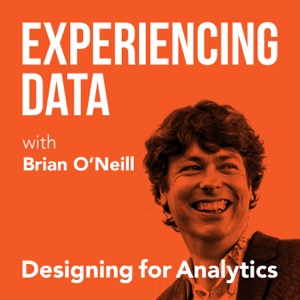
Categories:
Today I’m chatting with Josh Noble, Principal User Researcher at TruEra. TruEra is working to improve AI quality by developing products that help data scientists and machine learning engineers improve their AI/ML models by combatting things like bias and improving explainability. Throughout our conversation, Josh—who also used to work as a Design Lead at IDEO.org—explains the unique challenges and importance of doing design and user research, even for technical users such as data scientists. He also shares tangible insights on what informs his product design strategy, the importance of measuring product success accurately, and the importance of understanding the current state of a solution when trying to improve it. Highlights/ Skip to: Josh introduces himself and explains why it’s important to do design and user research work for technical tools used by data scientists (00:43) The work that TruEra does to mitigate bias in AI as well as their broader focus on AI quality management (05:10) Josh describes how user roles informed TruEra’s design their upcoming monitoring product, and the emphasis he places on iterating with users (10:24) How Josh approaches striking a balance between displaying extraneous information in the tools he designs vs. removing explainability (14:28) Josh explains how TruEra measures product success now and how they envision that changing in the future (17:59) The difference Josh sees between explainability and interpretability (26:56) How Josh decided to go from being a designer to getting a data science degree (31:08) Josh gives his take on what skills are most valuable as a designer and how to develop them (36:12) Quotes from Today’s Episode “We want to make machine learning better by testing it, helping people analyze it, helping people monitor models. Bias and fairness is an important part of that, as is accuracy, as is explainability, and as is more broadly AI quality.” — Josh Noble (05:13) “These two groups, the data scientists and the machine-learning engineer, they think quite differently about the problems that they need to solve. And they have very different toolsets. … Looking at how we can think about making a product and building tools that make sense to both of those different groups is a really important part of user experience.” – Josh Noble (09:04) “I’m a big advocate for iterating with users. To the degree possible, get things in front of people so they can tell you whether it works for them or not, whether it fits their expectations or not.” – Josh Noble (12:15) “Our goal is to get people to think about AI quality differently, not to necessarily change. We don’t want to change their performance metrics. We don’t want to make them change how they calculate something or change a workflow that works for them. We just want to get them to a place where they can bring together our four pillars and build better models and build better AI.” – Josh Noble (17:38) “I’ve always wanted to know what was going on underneath the design. I think it’s an important part of designing anything to understand how the thing that you are making is actually built.” – Josh Noble (31:56) “There’s a empathy-building exercise that comes from using these tools and understanding where they come from. I do understand the argument that some designers make. If you want to find a better way to do something, spending a ton of time in the trenches of the current way that it’s done is not always the solution, right?” – Josh Noble (36:12) “There’s a real empathy that you build and understanding that you build from seeing how your designs are actually implemented that makes you a better teammate. It makes you a better collaborator and ultimately, I think, makes you a better designer because of that.” – Josh Noble (36:46) “I would say to the non-designers who work with designers, measuring designs is not invalidating the designer. It doesn’t invalidate the craft of design. It shouldn’t be something that designers