108 - Google Cloud’s Bruno Aziza on What Makes a Good Customer-Obsessed Data Product Manager
Experiencing Data w/ Brian T. O’Neill (UX for AI Data Products, SAAS Analytics, Data Product Management) - A podcast by Brian T. O’Neill from Designing for Analytics - Tuesdays
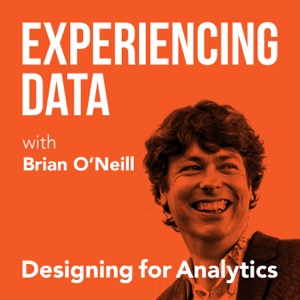
Categories:
Today I’m chatting with Bruno Aziza, Head of Data & Analytics at Google Cloud. Bruno leads a team of outbound product managers in charge of BigQuery, Dataproc, Dataflow and Looker and we dive deep on what Bruno looks for in terms of skills for these leaders. Bruno describes the three patterns of operational alignment he’s observed in data product management, as well as why he feels ownership and customer obsession are two of the most important qualities a good product manager can have. Bruno and I also dive into how to effectively abstract the core problem you’re solving, as well as how to determine whether a problem might be solved in a better way. Highlights / Skip to: Bruno introduces himself and explains how he created his “CarCast” podcast (00:45) Bruno describes his role at Google, the product managers he leads, and the specific Google Cloud products in his portfolio (02:36) What Bruno feels are the most important attributes to look for in a good data product manager (03:59) Bruno details how a good product manager focuses on not only the core problem, but how the problem is currently solved and whether or not that’s acceptable (07:20) What effective abstracting the problem looks like in Bruno’s view and why he positions product management as a way to help users move forward in their career (12:38) Why Bruno sees extracting value from data as the number one pain point for data teams and their respective companies (17:55) Bruno gives his definition of a data product (21:42) The three patterns Bruno has observed of operational alignment when it comes to data product management (27:57) Bruno explains the best practices he’s seen for cross-team goal setting and problem-framing (35:30) Quotes from Today’s Episode “What’s happening in the industry is really interesting. For people that are running data teams today and listening to us, the makeup of their teams is starting to look more like what we do [in] product management.” — Bruno Aziza (04:29) “The problem is the problem, so focus on the problem, decompose the problem, look at the frictions that are acceptable, look at the frictions that are not acceptable, and look at how by assembling a solution, you can make it most seamless for the individual to go out and get the job done.” – Bruno Aziza (11:28) “As a product manager, yes, we’re in the business of software, but in fact, I think you’re in the career management business. Your job is to make sure that whatever your customer’s job is that you’re making it so much easier that they, in fact, get so much more done, and by doing so they will get promoted, get the next job.” – Bruno Aziza (15:41) “I think that is the task of any technology company, of any product manager that’s helping these technology companies: don’t be building a product that’s looking for a problem. Just start with the problem back and solution from that. Just make sure you understand the problem very well.” (19:52) “If you’re a data product manager today, you look at your data estate and you ask yourself, ‘What am I building to save money? When am I building to make money?’ If you can do both, that’s absolutely awesome. And so, the data product is an asset that has been built repeatedly by a team and generates value out of data.” – Bruno Aziza (23:12) “[Machine learning is] hard because multiple teams have to work together, right? You got your business analyst over here, you’ve got your data scientists over there, they’re not even the same team. And so, sometimes you’re struggling with just the human aspect of it.” (30:30) “As a data leader, an IT leader, you got to think about those soft ways to accomplish the stuff that’s binary, that’s the hard [stuff], right? I always joke, the hard stuff is the soft stuff for people like us because we think about data, we think about logic, we think, ‘Okay if it makes sense, it will be implemented.’ For most of us, getting stuff done is through people. And people are emotional, how can yo